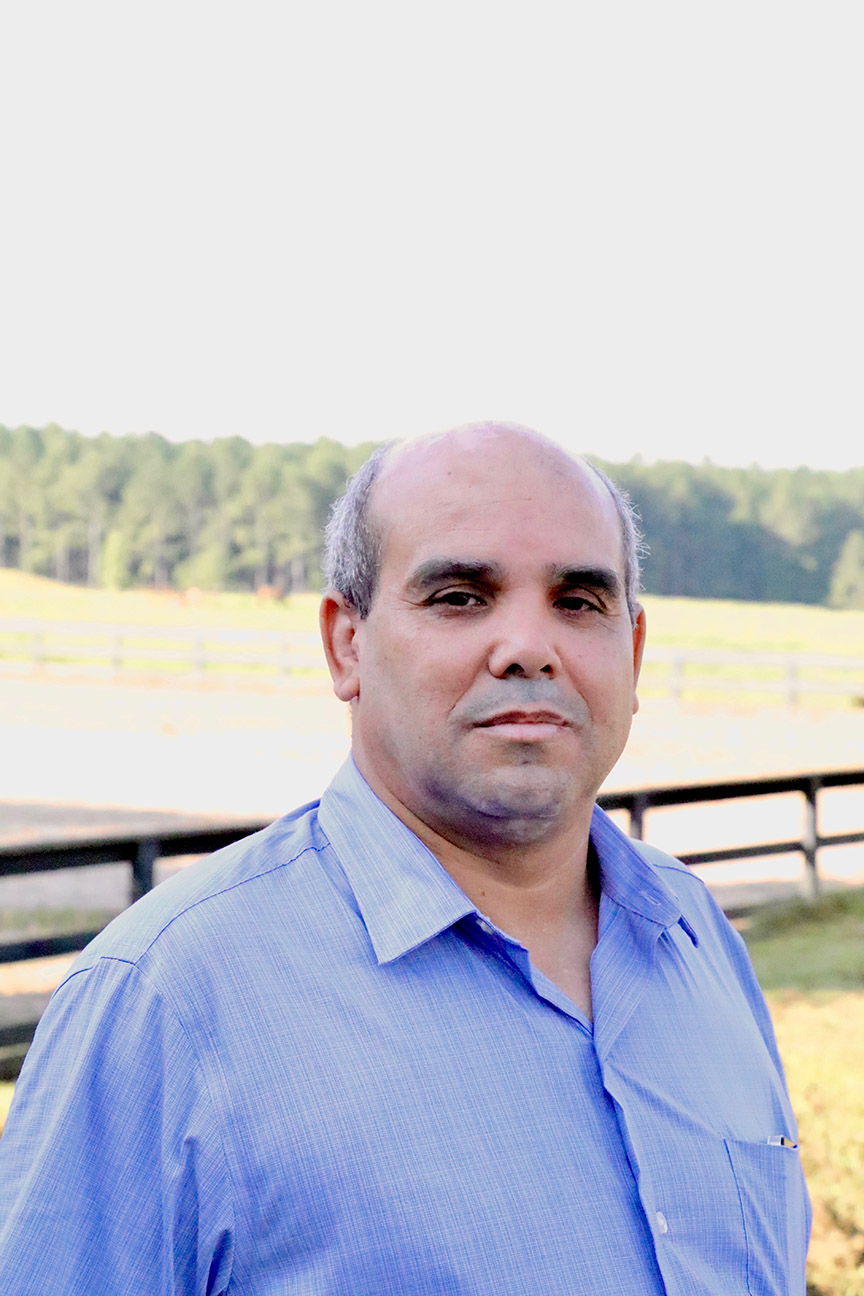
Own this profile? Request changes
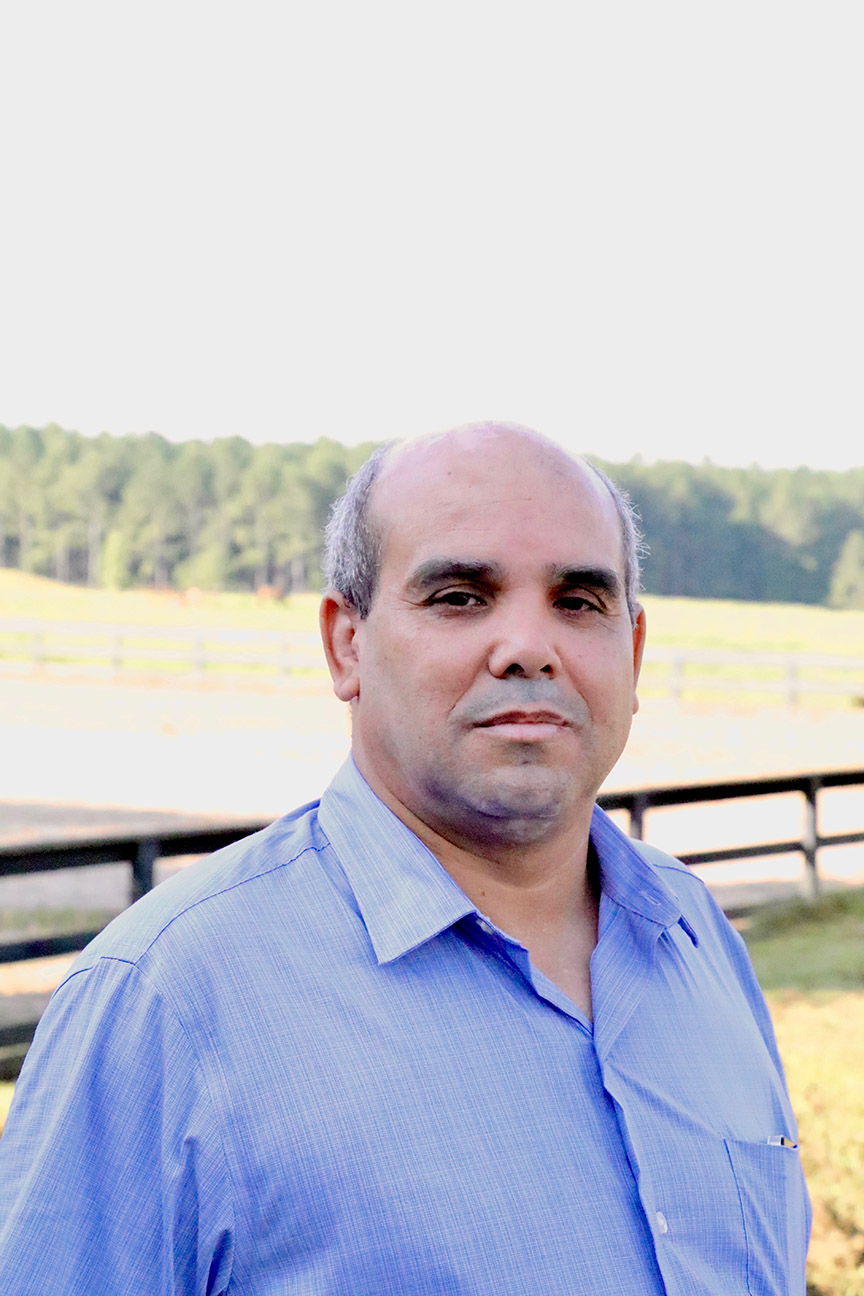
Biography
Dr. Rekaya is a Professor of Animal Breeding and Genetics at the University of Georgia. He received an agriculture engineer degree from the Hight Institute of Agriculture, Tunisia, a Master of Science from the International Center for Advanced Studies in Mediterranean Agriculture of Zaragoza, Spain, and a PhD from the Polytechnic University of Madrid, Spain.
Dr. Rekaya has an extensive experience in quantitative genetics, genomics, biostatistics and bioinformatics. His research program is centered in the development of statistical and computational tools for analysis for big genetic and genomic data sets with application in livestock, poultry and human. Dr. Rekaya’s research has been supported by several federal, state and private funding agencies including the USDA NRI, AFRI and NIFA programs, NAAB, the Georgia Agricultural Commodity Commission for beef, Cobbs-Vantress, Inc., Merial, Inc., and Tyson Foods. He has published 134 refereed journal articles, 4 book chapters, and over 170 abstracts and proceedings.
Dr. Rekaya is an adjunct professor at the Statistics department and an associate faculty member with the Institute of Bioinformatics at UGA. He has taught several international courses in Canada, Norway, Brazil and Kenya.
Agriculture Engineer, Ecole Superieur d’Agriculture de Mateur, Tunisia
M.S., International Center for Advanced Studies in Mediterranean Agriculture, Zaragoza, Spain
Ph.D., Polytechnic University of Madrid, Spain
Teaching
- ADSC8210 (3 hrs): ADVANCED STATISTICAL METHODS IN ANIMAL BREEDING – This course covers methods and techniques required for the implementation of full Bayesian inferences with emphasis towards applications in livestock and poultry genetic improvement programs. It covers theoretical and practical aspects of sampling of random variables, deriving full conditional distributions, and Bayesian implementation of mixed linear models via Markov Chain Monte Carlo Methods (Gibbs Sampling and Metropolis-Hasting algorithms)
- ADSC8000 (3 hrs): STATISTICAL GENETICS – This course covers topics related to QTL-mapping, genome-wide association studies (GWAS), genomic selection (GS), and technological and computational advances and their potential use in livestock and poultry genetic improvement programs.
- ADSC8886 (1-3 hrs): CURRENT LITERATURE IN GENETICS AND COMPUTING IN ANIMAL BREEDING - This is the animal breeding and genetics group seminar course (1 hr). Sometimes it is used to teach punctual critical needs (e.g., Analysis of discrete data with applications in animal breeding and genetics)
- ADSC 4960R: FACULTY-MENTORED UNDERGRADUATE RESEARCH I – Undergraduate students will be provided the opportunity to carry research at Dr. Rekaya’s Lab. Students will be involved in field data collection, data pre-processing, data analysis, and results dissemination through co-authorship of posters, abstracts, and journal articles. If you are interested in getting involved in research related to genetic solutions to horn fly problem in beef cattle, methane emissions, broiler water use, or the use of genomic information for animal selection, please email Dr. Rekaya (rrekaya@uga.edu)
Research
Current research projects at Dr. Rekaya’s Lab
- Developing a genetic solution to the billion-dollar horn fly problem: Dr. Rekaya and his team has been involved since 2020 in trying to find a solution for genetic selection of beef cattle for increased resistance/ tolerance for horn fly (a major problem costing the cattle industry over $1 billion a year). Classical and machine-based approaches are being used to developed count and non-count-based methods to assess fly abundance. Similarly, machine learning and advanced Bayesian predictive models are being developed to identity the threshold for the onset of economic injury (tolerance) and the decay after onset (resistance). Functional genomic analyses will be carried out to identify potential polymorphisms is association with the different horn fly abundance phenotypes. This research effort is funded by the Georgia Beef Commission and USDA-NIFA. If you are interested in an internship or to carry a faculty-mentored undergraduate research, please contact Dr. Rekaya at rrekaya@uga.edu
- Integrating single nucleotide polymorphisms (SNPs) level inbreeding in breeding values prediction: The aim of this project is to develop to development statistical models for better utilization of single nucleotide polymorphisms (SNPs) in predicting expected progeny differences in beef cattle. Developing new methods to assess inbreeding using genomic information and the better understanding of ancient and recent inbreeding will provide additional tools to a more precise estimation of inbreeding depression resulting in a more accurate estimation of genetic merit. This project is funded by the USDA-ARS.
- Improving the efficiency of water intake utilization in poultry: This a collaborative project between Dr. Rekaya and Dr. Samuel E. Aggrey (Poultry Science) Labs. The project aims at develop practical genetic and molecular signatures that could be used to select and or screen broiler chickens based on their efficiency in water utilization without compromising feed efficiency and growth traits. Molecular and genomic approaches are used to identify relevant variants and biomarkers associated with water use efficiency. This project is funded by the National Academy of Sciences
- Examine associations of factors related to greenhouse gas emissions and feed efficiency in Jersey and Holstein dairy cows: The overall goal of this project is to increase the competitiveness and sustainability of Georgia dairy industry through the reduction of greenhouse gas (GHG) emissions and the increase of feed efficiency. This is a multi-disciplinary research project aiming at understanding the associations between GHG emission, feed efficiency, gut microbiota, digestibility, and behavior of lactating Holstein and Jersey dairy cows. This a collaboration effort with Drs Tao (PI), Rekaya (Co-PI), Bohlen (Co-PI), and Ryman (Co-PI). The project is funded by the Georgia Beef Commission and the Institute for Integrative and Precision Agriculture
Students and visitors at Dr. Rekaya Lab
- Amanda Warner, PhD student
- Mahsa Zare, PhD student
- Koushik Das, PhD student
- Evan Hartono, PhD student
- Grady Cheek, Undergraduate student
- Jaden Hallman, Undergraduate student
- Narjice Chafai, Fulbright scholar
- Yacouba Zare, Scientist, Burkina Faso
Select referred journal articles (out of a total of 182 as of January 2024)
1. Rekaya R., M. J. Carabano and M. A. Toro, 2000. Assessment of heterogeneity of residual variances using changepoints techniques. Genet. Sel. Evol. 32(4):383-394.
2. Rekaya, R., M. J. Carabano, M. A. Toro, 2000. Bayesian analysis of lactation curves of Holstein-Friesian cattle using nonlinear models. J. Dairy Sci. 83:2691-2701.
3. Rekaya R., K. Weigel and D. Gianola, 2001. Application of a structural model for genetic covariances in International Dairy evaluations. J. Dairy Sci. 84:1525-1530.
4. Rekaya R., K. Weigel and D. Gianola, 2001. Threshold model misclassified binary responses with applications to animal breeding. Biometrics 57: 1123-1129
5. Donoghue, K. A., R. Rekaya, and J. K. Bertrand, 2004. Comparison of methods for handling censored records in beef fertility data: Field data. J. Anim. Sci. 82:357-361.
6. Sapp, R. L., R. Rekaya, I. Misztal, and T. Wing. 2004. Male and female fertility and hatchability in chickens: A longitudinal mixed model approach. Poult. Sci. 83:1253-1259.
7. Sapp, R. L., M. L. Spangler, R. Rekaya, and J. K. Bertrand. 2005. A simulation study for analysis of uncertain binary responses: Application to first insemination success in beef cattle. Genet. Sel. Evol. 37:615-634.
8. Zhang, W., R. Rekaya, and J. K. Bertrand. 2005. A Method for Predicting Disease Subtypes in Presence of Misclassification Among Training Samples Using Gene Expression Data: Application to Human Breast Cancer. Bioinformatics. 22:317-325
9. Robbins, K. R., Zhang, W., J. K. Bertrand, R. Rekaya. 2008. Ant colony optimization for feature selection in high dimensionality data sets. Math Med Biol. 24(4):413-426.
10. Spangler, M. L., K. R. Robbins, J. K. Bertrand, M. MacNeil, and R. Rekaya. 2008. Ant colony optimization as a method for strategic genotype sampling. Animal Genetics 40: 308 – 314.
11. Joseph, S., K. Robbins., W. Zhang., R. Rekaya. 2008. Effects of misdiagnosis in input data on the identification of differential expression genes in incipient Alzheimer patients. In Silico Biology. 8: 41-48.
12. Spangler M. L., R. L. Sapp, J. K. Bertrand, M. D. MacNeil, and R. Rekaya. 2008. Different methods of selecting animals for genotyping to maximize the amount of genetic information known in the population. J. Anim. Sci. 86:2471-2479
13. Wang, Y., and R. Rekaya. (2009) A comprehensive analysis of gene expression evolution between humans and mice. Evolutionary Bioinformatics 5: 81-90.
14. Spangler M L; K. R. Robbins, J. K. Bertrand, M. Macneil, and R. Rekaya. 2009.Ant colony optimization as a method for strategic genotype sampling. Animal Genetics. 40(3):308-14.
15. Wang, Y., and R. Rekaya. (2010). LSOSS: Detection of Cancer Outlier Differential Gene Expression. Biomarker Insights, 5: 69–78.
16. Wang, Y., K. Robbins and R. Rekaya. (2010). Comparison of computational models for assessing conservation of gene expression across species. PLoS ONE 2010 8: 5(10) :e13239
17. Wang Y., X. Liu, K. Robbins, and R. Rekaya (2010). AntEpiSeeker: detecting epistatic interactions for case-control studies using a two-stage ant colony optimization algorithm. BMC Res Notes, 3: 117.
18. Zhang W, K. Robbins, Y. Wang, K. Bertrand and R. Rekaya. (2010). A jackknife-like method for classification and uncertainty assessment of multi-category tumor samples using gene expression information. BMC Genomics 11: 273.
19. Joseph SJ, Robbins KR, Zhang W and Rekaya R. (2010). Comparison of Two Output-Coding Strategies for Multi-Class Tumor Classification Using Gene Expression Data and Latent Variable Model as Binary Classifier. Cancer Informatics, 9: 39-48.
20. Rekaya, R., R. Sapp, EH. Hay, R. Davis, and K. Bertrand. 2011. Simulation Study for Analysis of Binary Responses in the Presence of Extreme Case Problems. Genetics Selection Evolution. Genet. Sel. Evol. 43:41.
21. Liu, Y., Y. Wang, R. Rekaya and TN. Sriran. (2012). Sample size determination for classifiers based on single-nucleotide polymorphisms. Biostatistics, 13(2): 217-227.
22. Wang, Y., S.J. Joseph, X. Liu, M. Kelly, and R. Rekaya. 2012. SNPxGE2: a database for human SNP-coexpression associations. Bioinformatics, 28(3): 403-410.
23. Rekaya, R., R. L. Sapp, T. Wing, and S. aggrey. 2013. Genetic evaluation for growth, body composition, feed efficiency, and leg soundness. Poult. Sci. 92:923-929
24. Smith, S., EH Hay, N. Farhat, and R. Rekaya. 2013. Genome wide association studies in presence of misclassified binary responses. BMC Genetics 14:124
25. Rekaya, R. and S. Aggrey. (2015). Genetic properties of residual feed intakes for maintenance and growth, and the implications of error measurement. J. anim. Sci. 93:944-948
26. Rekaya, R., Smith, S., E. H. Hay, A. Ling, S. Aggrey. 2016. Analysis of discrete responses with error specific misclassification. The application of Clinical genetics, 9:169-177
27. Chang, L. Y., S. Toghiani, A. Ling, E. H. Hay, S. E. Aggrey, R. Rekaya. (2017). Analysis of Multiple Binary Responses Using a Threshold Model. Journal of Agricultural Biological and Environmental Statistics 22:640-651
28. Sumreddee, P., S.Togniani, E. H.i Hay, A. Roberts, S. E. Aggrey, and R. Rekaya (2018). Inbreeding depression in line 1 Hereford cattle population using pedigree and genomic information. J Anim Sci 97(1):1-18, https://doi.org/10.1093/jas/sky385
29. Chang, L.Y., S.Toghiani, A. Ling, S. E. Aggrey,and R. Rekaya. (2018). High density marker panels, SNPs prioritizing and accuracy of genomic selection. BMC Genet. 9: 4. https://doi.org/10.1186/s12863-017-0595-2
30. Ling A, EH Hay, SE Aggrey, and R. Rekaya. 2018. A Bayesian approach for analysis of ordered categorical responses subject to misclassification. PLoS One 13(12): e0208433 2018
31. Chang, L. Y., Toghiani, S., Hay, E. H., Aggrey, S. E., & Rekaya, R. (2019). A Weighted Genomic Relationship Matrix Based on Fixation Index (FST) Prioritized SNPs for Genomic Selection. Genes, 10(11), 922. doi:10.3390/genes10110922
32. Glenn, T. C., R. Nilsen, T. J. Kieran, J. W. Finger Jr., T. W. Pierson, K. E. Bentley, S. L. Hoffberg, S. Louha, F. J. Garcia-De Leon, M. A. D. R. Portilla, K. D. Reed, J. L. Anderson, J. K. Meece, S. E. Aggrey, R. Rekaya, M. Alabady, M. Belanger, K. Winker, and B. C. Faircloth.2019. Adapterama I: Universal stubs and primers for thousands of dual-indexed Illumina libraries (iTru & iNext). PeerJ, 7, e7755. doi:10.7717/peerj.7755
33. Chang, L. -Y., Toghiani, S., Aggrey, S. E., & Rekaya, R. (2019). Increasing accuracy of genomic selection in presence of high-density marker panels through the prioritization of relevant polymorphisms. BMC Genet, 20(1), 21. doi:10.1186/s12863-019-0720-5
34. Sumreddee, P., Toghiani, S., Hay, E. H., Roberts, A., Aggrey, S. E., & Rekaya, R. (2019). Inbreeding depression in line 1 Hereford cattle population using pedigree and genomic information. J. Anim. Sci. 97(1), 1-18. doi:10.1093/jas/sky385
35. Wang, Z., J. Zhou, B. Marshall, R. Rekaya, K. Ye, H. Liu. (2020). SARS-CoV-2 receptor ACE2 is enriched in a subpopulation of mouse tongue epithelial cells in non-gustatory papillae, but not in taste buds or embryonic oral epithelium. ACS Pharmacology & Translational Science, 3(4):749-758
36. Sumreddee, P., S. Toghiani, E. H. Hay, A. Roberts, S. E. Aggrey, and R. Rekaya. (2021). Grid search approach to discriminate between old and recent inbreeding using pedigree and genomic information. BMC Genomics 22, 538 (2021). https://doi.org/10.1186/s12864-021-07872-z
37. Ling, A.S., E.H. Hay, S.E. Aggrey and R. Rekaya. (2021). Dissection of the impact of prioritized QTL-linked and -unlinked SNP markers on the accuracy of genomic selection. BMC Genomics. 22:1-14. https://doi.org/10.1186/s12863-021-00979-y
38. Sumreddee, P., Hay, E. H., Toghiani, S., Roberts, A., Aggrey, S. E., & Rekaya, R. 2021. Grid search approach to discriminate between old and recent inbreeding using phenotypic, pedigree and genomic information. BMC Genomics, 22(1). doi:10.1186/s12864-021-07872-z
39. Warner, A., Ling, A., Krause, T., Heins, B., Hinkle, N., Pringle, D., S. E. Aggrey, and R. Rekaya. 2022. Thrombin as a Potential Proxy to Select for Horn Fly Abundance in Beef Cattle. Animals, 12(21), 2982. doi:10.3390/ani12212982
40. Ling, A., R. H. Hay, S. E. Aggrey, and R. Rekaya. 2022. Fuzzy Logic as a Strategy for Combining Marker Statistics to Optimize Preselection of High-Density and Sequence Genotype Data. Genes, 13(11), 2100. doi:10.3390/genes13112100
41. Warner, A. M., B. D. Heins, N. C. Hinkle, T. D. Pringle, S. E. Aggrey, and R. Rekaya. 2023. Genetic parameters of subjective and image-based horn fly abundance phenotypes. Frontiers in Animal Science, 4. doi:10.3389/fanim.2023.1284684